A Time Series Model to Forecast COVID-19 Infection rate in Nigeria Using Box-Jenkins Method
DOI:
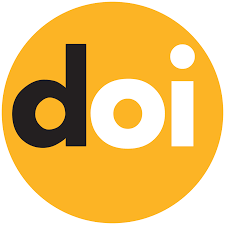
Keywords:
COVID-19, ARIMA, Forecast, Autocorrelation, Partial AutocorrelationAbstract
Coronavirus declared as a global pandemic by WHO has emerged as the most aggressive disease negatively affecting over 90% of the countries in the world. Nigeria, one of the most populated countries in Africa is not an exception. This study focuses on analyzing the intrinsic patterns in the COVID-19 spread in Nigeria using the Box-Jenkins procedure. Data of daily confirmed cases of COVID-19 in Nigeria was retrieved from Nigeria Centre for Disease Control (NCDC) official website from February 27, 2020 to October 31, 2020 to identify the series components, estimate parameters, develop an appropriate stochastic predictive model and use the model to forecast future trend of the deadly virus. The Autoregressive Integrated Moving Average (ARIMA) of order (0,1,1) was identified as the most suitable model based on the analysis of the autocorrelation (ACF), partial autocorrelation functions (PACF) and Akaike Information Correction (AICc) value. R software version 4.0.3 was used to analyze the trend which moothen the series by using 8-point moving average to extract the irregular component as wellas differencing the series one step further to obtain a stationary series. We performed the Augmented Dickey-Fuller Unit root test, parameter estimation and Ljung-Box test to check the proposed model’s conformity to the stationary univariate process. A 85 – day (1st Oct., 2020 – 24th Jan., 2020) forecast shows a gradual decline in the successive number of confirmed cases of infection indicating the effectiveness of the intervention strategies employed by the Task Force to contain the virus. The concerned authorities can apply the forecasted trend to make further informed decisions on the measures to be put in place to reduce diffusion of the deadly virus into the country.
Downloads
Published
How to Cite
Issue
Section
License
Copyright (c) 2021 CA Ortese, TG Ieren, AJ Tamber

This work is licensed under a Creative Commons Attribution-ShareAlike 4.0 International License.